RAPSIM
Target Classification
RAPSIM Target Classification
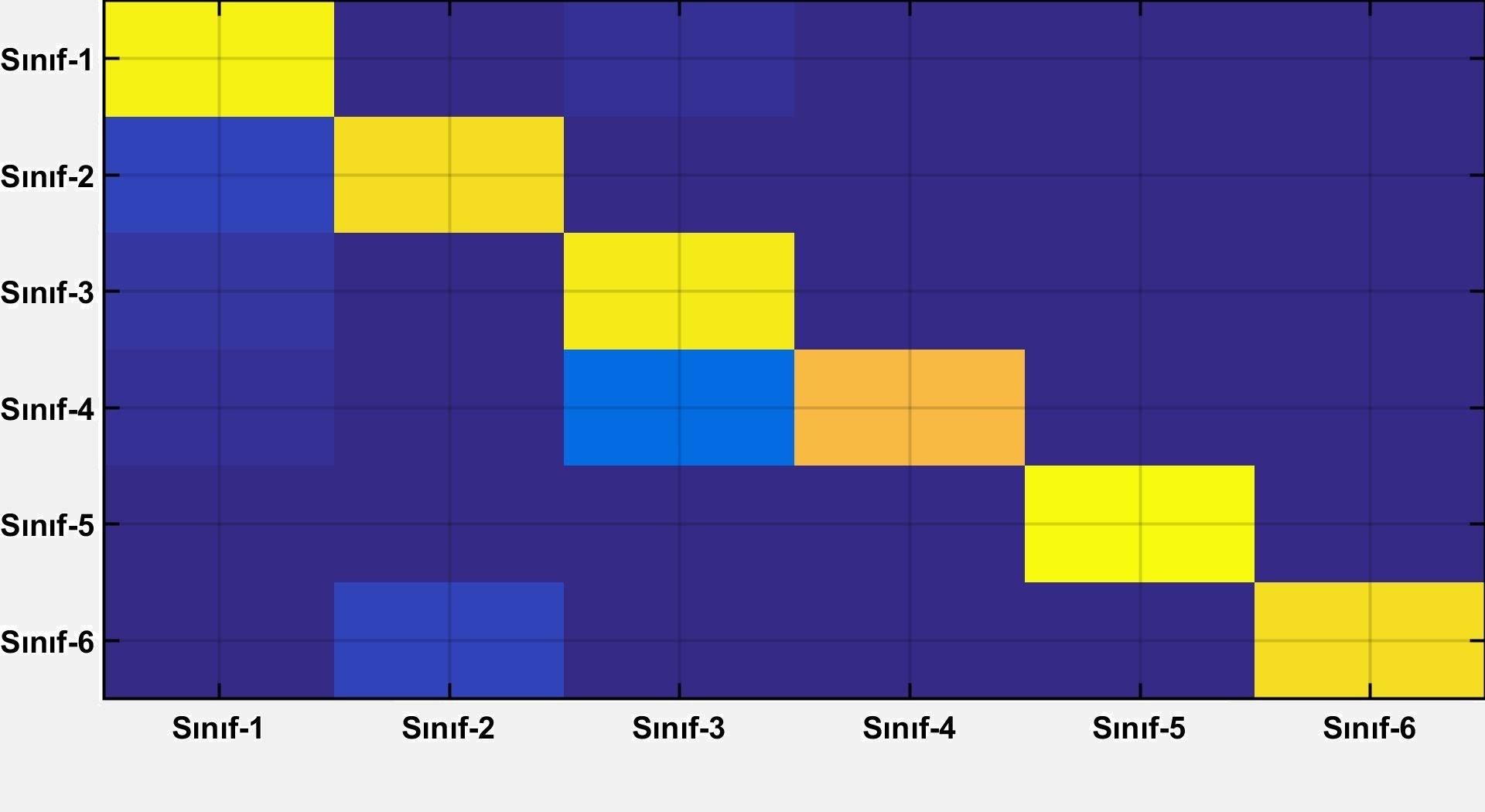
RAPSIM Target Classification conducts classification activities for land, air, or sea platforms using measurement and/or synthetic data obtained from radar, sonar, or optical sensors. In this process, various features and different classification structures are employed to analyze the characteristic properties and behaviors of targets, allowing for the differentiation between classes of objects. Target classification plays a critical role in target detection and threat assessment in defense systems.
Target Types
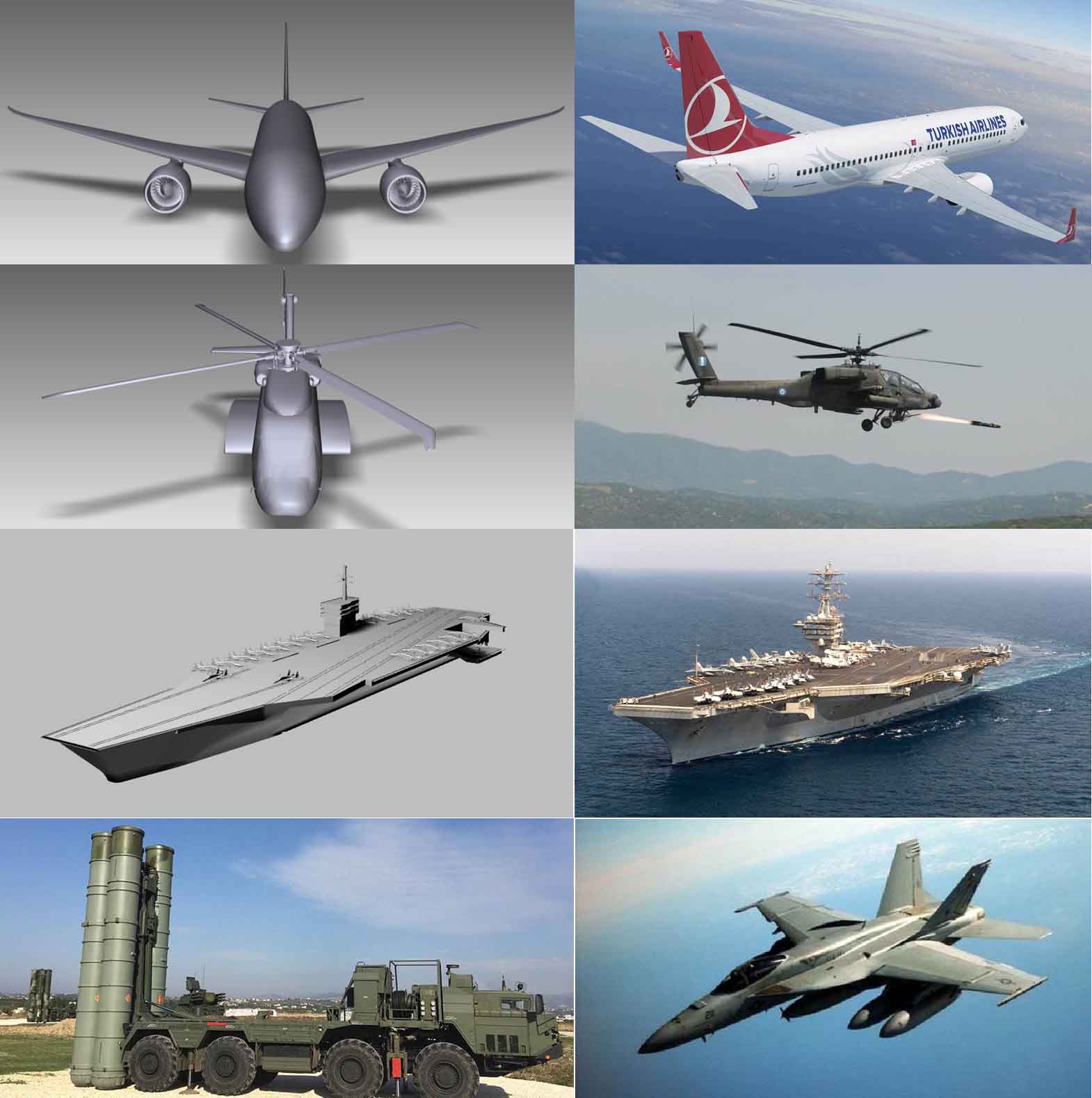
Input Data Types
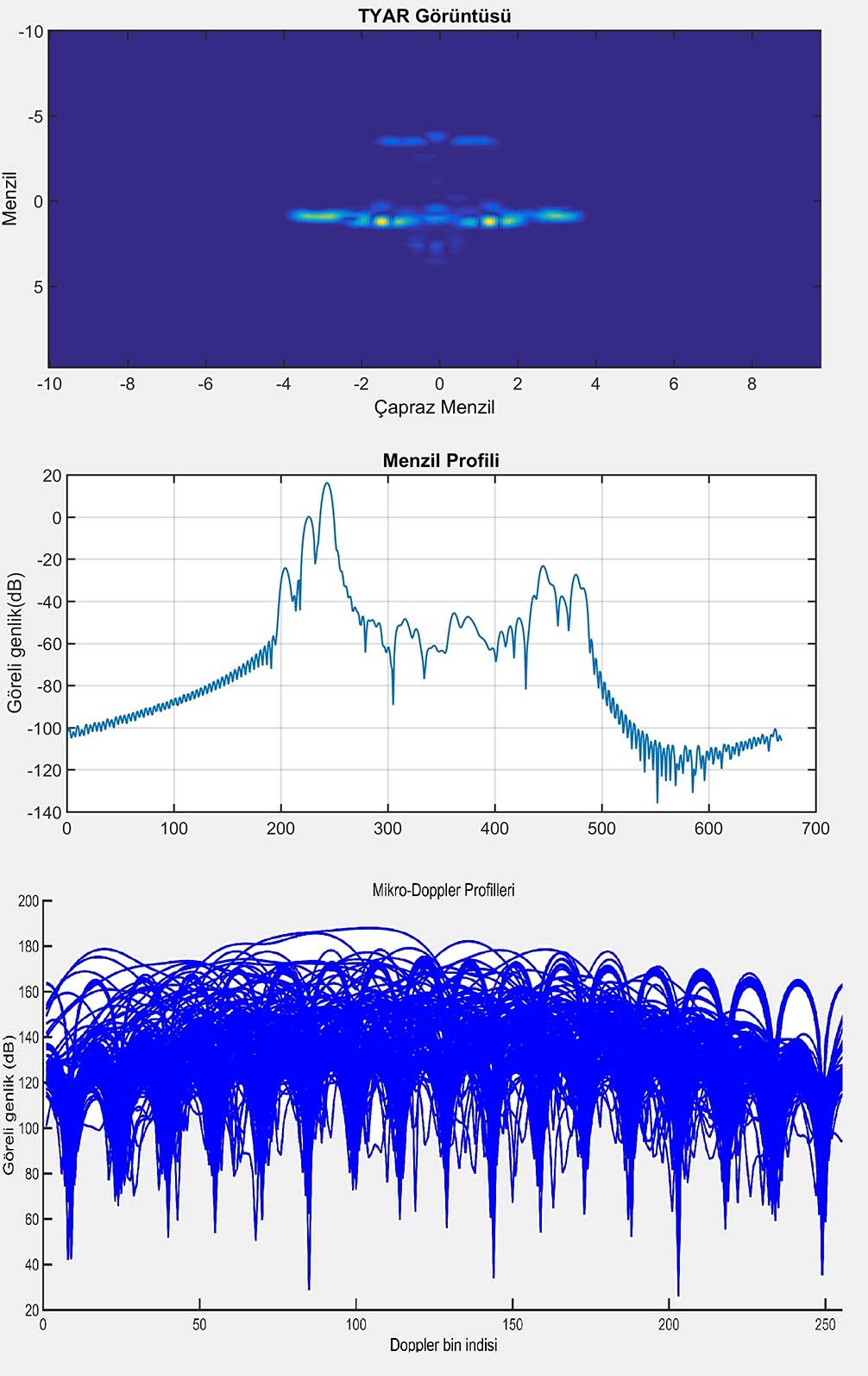
Feature Types
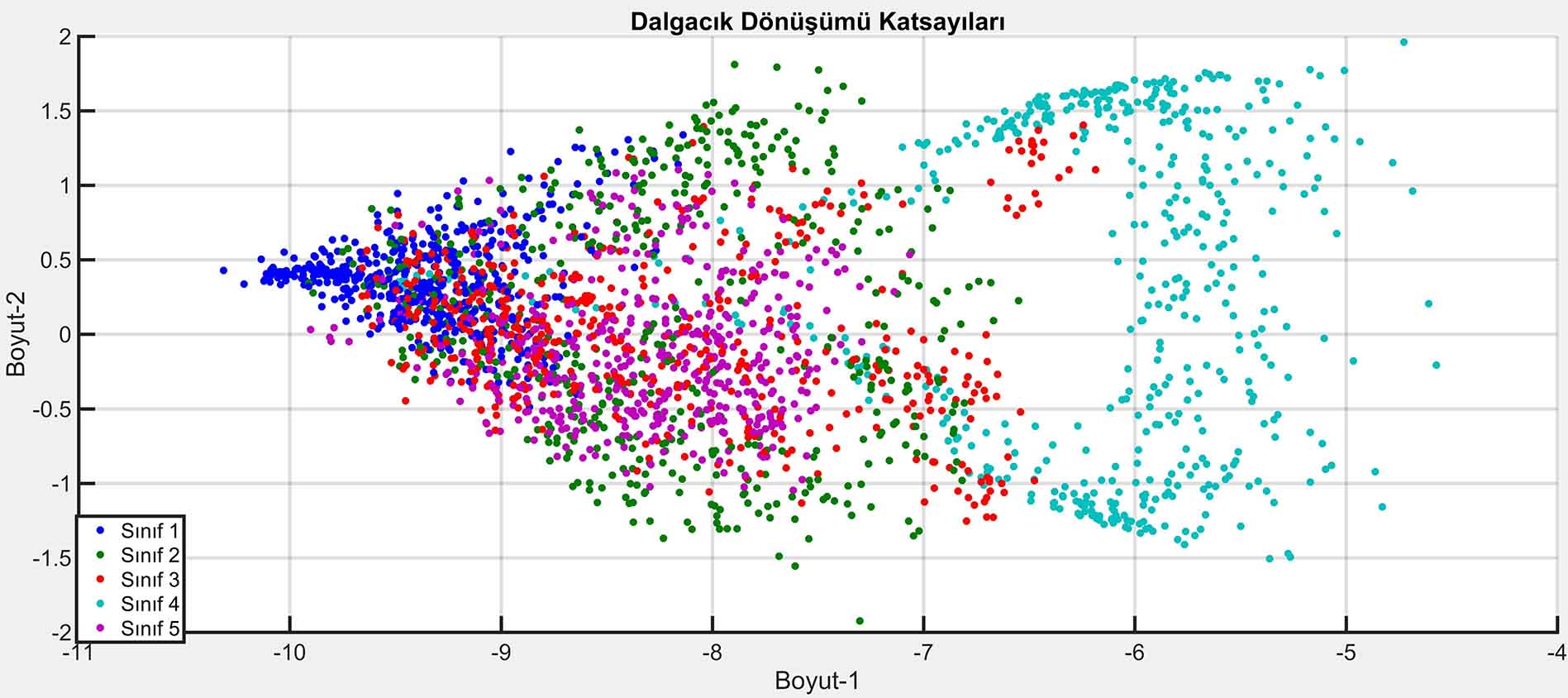
Classifier Types
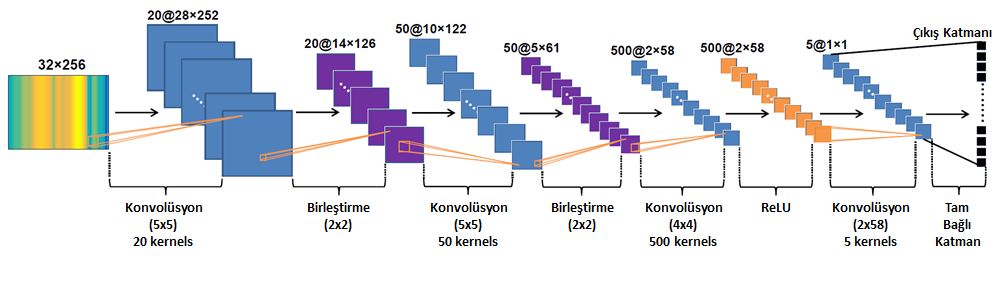
In classification studies, a variety of classifier structures can be applied to achieve the highest recognition performance, either individually or on an average basis. These structures include basic Bayesian, proximity-based (k-NN), cost function optimization (perceptron, least squares, support vector machines, artificial neural networks), or deep learning (convolutional neural networks) approaches.